
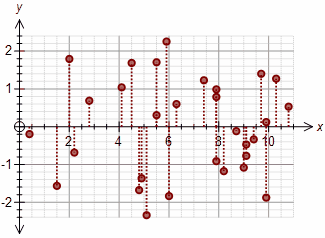
Thus, the residual for this data point is 60 – 60.797 = -0.797. Thus, the predicted height of this individual is: To find out the predicted height for this individual, we can plug their weight into the line of best fit equation: The first individual has a weight of 140 lbs. Example 1: Calculating a Residualįor example, recall the weight and height of the seven individuals in our dataset:

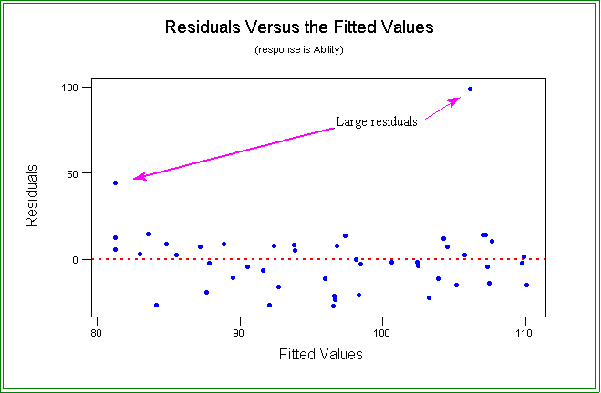
For each data point, we can calculate that point’s residual by taking the difference between it’s actual value and the predicted value from the line of best fit. This difference between the data point and the line is called the residual. Notice that the data points in our scatterplot don’t always fall exactly on the line of best fit:
#Residual graph how to
Height = 32.783 + 0.2001*(weight) How to Calculate Residuals In this example, the line of best fit is: Where ŷ is the predicted value of the response variable, b 0 is the y-intercept, b 1 is the regression coefficient, and x is the value of the predictor variable. The formula for this line of best fit is written as: Using linear regression, we can find the line that best “fits” our data: If we graph these two variables using a scatterplot, with weight on the x-axis and height on the y-axis, here’s what it would look like:įrom the scatterplot we can clearly see that as weight increases, height tends to increase as well, but to actually quantify this relationship between weight and height, we need to use linear regression. Let weight be the predictor variable and let height be the response variable. The other variable, y, is known as the response variable.įor example, suppose we have the following dataset with the weight and height of seven individuals: One variable, x, is known as the predictor variable. Simple linear regression is a statistical method you can use to understand the relationship between two variables, x and y.
